C# Sorcery: Building a Chess Eninge for Your Magical Chessboard!
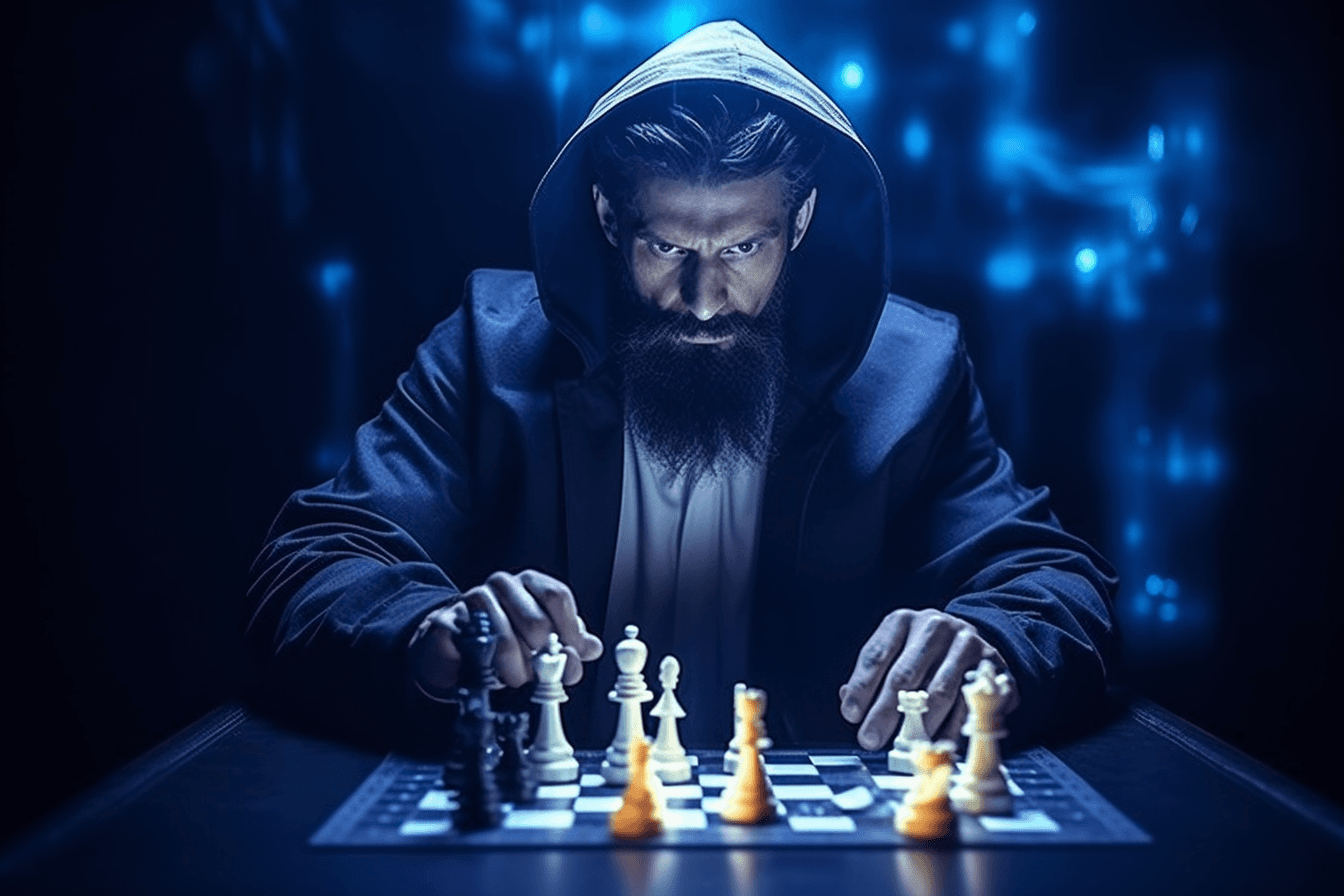
Welcome to the world of algorithms! If you're a beginner, fear not. In this guide, we'll navigate through the basics of algorithms, demystifying complex concepts and exploring practical examples.
1. The Landscape of Algorithms
Algorithms form the backbone of computer science. Let's embark on a journey to understand their landscape.
1.1 Decoding Big O Notation
One of the first challenges in understanding algorithms is grappling with Big O notation. It's like learning a new language, but fear not! We'll break it down:
def linear_search(arr, target):
for element in arr:
if element == target:
return True
return False
In this snippet, we have a simple linear search algorithm. Understanding its Big O complexity is key to evaluating its efficiency.
2. Practical Application
Theory without practice is like a recipe without cooking. Let's apply our knowledge to real-world scenarios.
2.1 Sorting Algorithms
Sorting is a fundamental operation. Here's a glimpse of a bubble sort implementation in Python:
def bubble_sort(arr):
n = len(arr)
for i in range(n):
for j in range(0, n-i-1):
if arr[j] > arr[j+1]:
arr[j], arr[j+1] = arr[j+1], arr[j]
2.2 Search Algorithms
Searching for information efficiently is crucial. Binary search is a classic algorithm to achieve this:
def binary_search(arr, target):
low, high = 0, len(arr) - 1
while low <= high:
mid = (low + high) // 2
if arr[mid] == target:
return mid
elif arr[mid] < target:
low = mid + 1
else:
high = mid - 1
return -1
3. Algorithmic Strategies
Algorithmic problem-solving requires strategic thinking. Let's explore some powerful strategies.
3.1 Recursion
Recursion is a powerful technique. Consider the classic example of calculating the factorial of a number:
def factorial(n):
if n == 0 or n == 1:
return 1
else:
return n * factorial(n-1)
3.2 Dynamic Programming
Dynamic programming can optimize solutions to overlapping subproblems. Here's an example with the Fibonacci sequence:
def fibonacci(n, memo={}):
if n in memo:
return memo[n]
if n <= 2:
return 1
memo[n] = fibonacci(n-1) + fibonacci(n-2)
return memo[n]
4. Algorithmic Challenges
Challenge yourself! Here are some algorithmic exercises to sharpen your problem-solving skills:
- Challenge 1: Implement a quicksort algorithm in your language of choice.
- Challenge 2: Solve the traveling salesman problem using a greedy algorithm.
5. Conclusion: A Foundation for Growth
Congratulations! You've embarked on a journey through the basics of algorithms. Whether you're a graphic designer exploring programming or a seasoned developer reinforcing your fundamentals, this beginner's guide provides a solid foundation for growth.
Ready to dive into the world of algorithms? Let's get started! For more in-depth information and code example wait for future posts!